Quantum computing companies are poised to exceed $1 billion in revenues by the close of 2025, to McKinsey & Company, underscoring how today’s quantum computers are already delivering customer value in their current phase of development.
This figure is projected to reach upwards of $37 billion by 2030, rising in parallel with escalating demand, as well as with the scale of the machines and the complexity of problem sets of which they will be able to address.
Several systems on the market today are fault-tolerant by design, meaning they are capable of suppressing error-causing noise to yield reliable calculations. However, the full potential of quantum computing to tackle problems of true industrial relevance, in areas like medicine, energy, and finance, remains contingent on an architecture that supports a fully fault-tolerant universal gate set with repeatable error correction—a capability that, until now, has eluded the industry.
ҹɫֱ is the first—and only—company to achieve this critical technical breakthrough, universally recognized as the essential precursor to scalable, industrial-scale quantum computing. This milestone provides us with the most de-risked development roadmap in the industry and positions us to fulfill our promise to deliver our universal, fully fault-tolerant quantum computer, Apollo, by 2029.
In this regard, ҹɫֱ is the first company to step from the so-called “NISQ” (noisy intermediate-scale quantum) era towards utility-scale quantum computers.
Unpacking our achievement: first, a ‘full’ primer
A quantum computer uses operations called gates to process information in ways that even today’s fastest supercomputers cannot. The industry typically refers to two types of gates for quantum computers:
- Clifford gates, which can be easily simulated by classical computers, and are relatively easy to implement; and
- Non-Clifford gates, which are usually harder to implement, but are required to enable true quantum computation (when combined with their siblings).
A system that can run both gates is classified as and has the machinery to tackle the widest range of problems. Without non-Clifford gates, a quantum computer is non-universal and restricted to smaller, easier sets of tasks - and it can always be simulated by classical computers. This is like painting with a full palette of primary colors, versus only having one or two to work with. Simply put, a quantum computer that cannot implement ‘non-Clifford’ gates is not really a quantum computer.
A fault-tolerant, or error-corrected, quantum computer detects and corrects its own errors (or faults) to produce reliable results. ҹɫֱ has the best and brightest scientists dedicated to keeping our systems’ error rates the lowest in the world.
For a quantum computer to be fully fault-tolerant, every operation must be error-resilient, across Clifford gates and non-Clifford gates, and thus, performing “a full gate set” with error correction. While some groups have performed fully fault-tolerant gate sets in academic settings, these demonstrations were done with only a few qubits and —too high for any practical use.
Today, we have published that establishes ҹɫֱ as the first company to develop a complete solution for a universal fully fault-tolerant quantum computer with repeatable error correction, and error rates low enough for real-world applications.
This is where the magic happens
The describes how scientists at ҹɫֱ used our System Model H1-1 to perfect magic state production, a crucial technique for achieving a fully fault-tolerant universal gate set. In doing so, they set a record magic state infidelity (7x10-5), 10x better than any .
Our simulations show that our system could reach a magic state infidelity of 10^-10, or about one error per 10 billion operations, on a larger-scale computer with our current physical error rate. We anticipate reaching 10^-14, or about one error per 100 trillion operations, as we continue to advance our hardware. This means that our roadmap is now derisked.
Setting a record magic state infidelity was just the beginning. The paper also presents the first break-even two-qubit non-Clifford gate, demonstrating a logical error rate below the physical one. In doing so, the team set another record for two-qubit non-Clifford gate infidelity (2x10-4, almost 10x better than our physical error rate). Putting everything together, the team ran the first circuit that used a fully fault-tolerant universal gate set, a critical moment for our industry.
Flipping the switch
In the , co-authored with researchers at the University of California at Davis, we demonstrated an important technique for universal fault-tolerance called “code switching”.
Code switching describes switching between different error correcting codes. The team then used the technique to demonstrate the key ingredients for universal computation, this time using a code where we’ve previously demonstrated full error correction and the other ingredients for universality.
In the process, the team set a new record for magic states in a distance-3 error correcting code, over 10x better than with error correction. Notably, this process only cost 28 qubits . This completes, for the first time, the ingredient list for a universal gate setin a system that also has real-time and repeatable QEC.
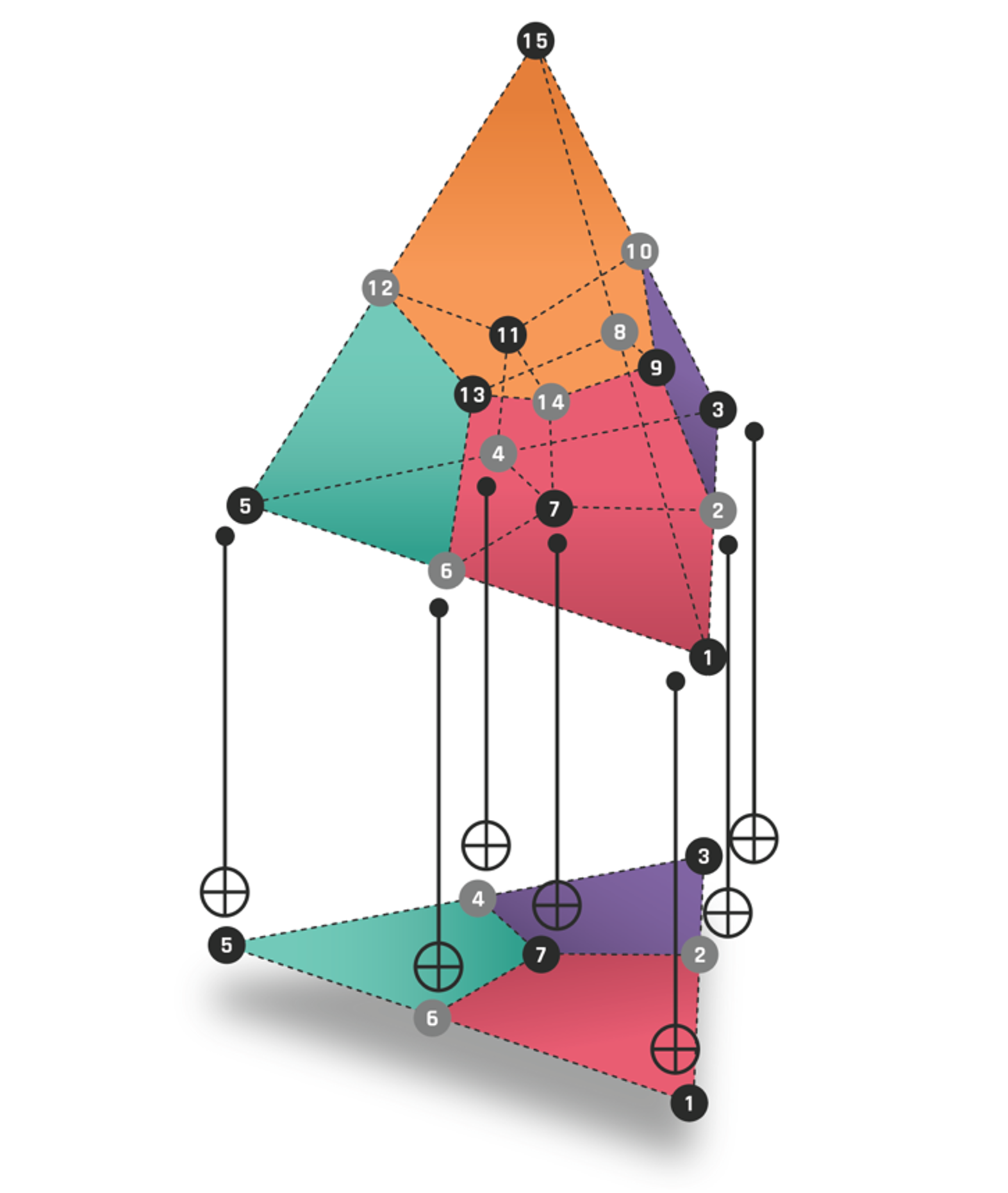
Fully equipped for fault-tolerance
Innovations like those described in these two papers can reduce estimates for qubit requirements by an order of magnitude, or more, bringing powerful quantum applications within reach far sooner.
With all of the required pieces now, finally, in place, we are ‘fully’ equipped to become the first company to perform universal fully fault-tolerant computing—just in time for the arrival of Helios, our next generation system launching this year, and what is very likely to remain as the most powerful quantum computer on the market until the launch of its successor, Sol, arriving in 2027.